SERENA WANG, “PROMISES AND PITFALLS OF MACHINE LEARNING FOR EDUCATION”
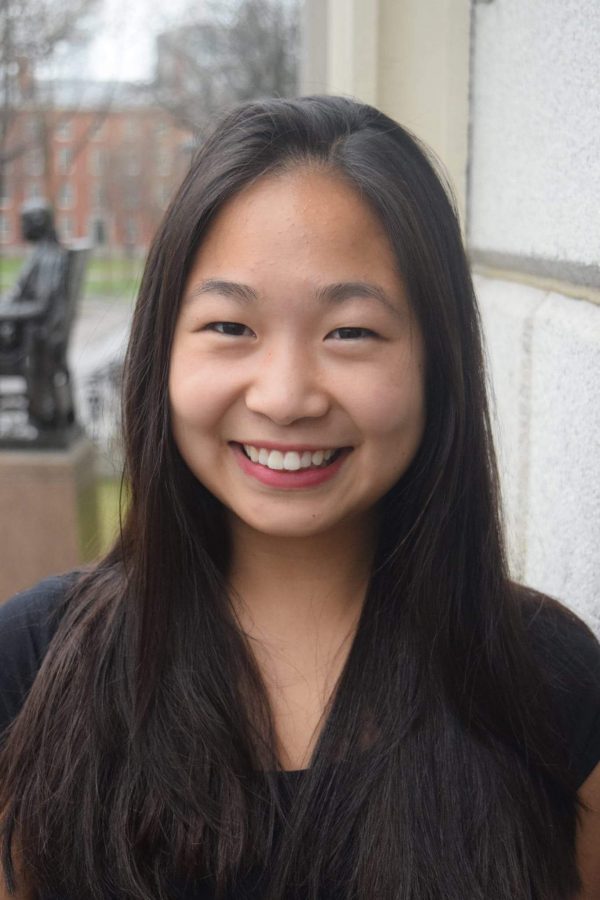
Oct. 28 (Thursday), 12:00: Serena Wang (PhD Candidate, UC Berkeley) presents as part of our series, Biased AI, on Promises and Pitfalls of Machine Learning for Education
—> Zoom Registration: https://uncc.zoom.us/meeting/register/tJ0lcO6pqDIiGNa0xqhRX6WOZuRaiBnoThKd
Abstract: Machine learning (ML) techniques are prevalent in the education sphere, from their use in MOOCs, to admissions, to predicting student dropout. Recently, public institutions faced controversy for high-profile applications such as GRADE, used in graduate computer science admissions at the University of Texas, and predictive analytics for forecasting A-level grades in the UK, because they exacerbate existing inequalities. With these highly-publicized failures — and the rapid proliferation of ML technologies accelerated in part by the COVID-19 pandemic — an urgent need exists to investigate how ML supports holistic education principles and goals. In this talk, I will present results from a qualitative study based on interviews with education domain experts, grounded in ML for education papers published in several highly-regarded ML conferences over the past decade. Our central research goal is to critically examine whether the stated or implied societal objectives of these papers are aligned with the ML problem formulation, objectives, and interpretation of results. This work joins a growing number of meta-analytical studies as well as critical analyses of the societal impact of ML. Specifically, this work fills a cross-disciplinary gap between the prevailing technical understanding of machine learning and the perspective of education researchers working with students and in policy.
About the series: Artificial Intelligence (AI) systems are poised to offer potentially revolutionary changes to fields as diverse as healthcare and traffic systems. However, there is a growing concern both that deployment of AI systems is increasing social power asymmetries and that ethical attention to those asymmetries requires going beyond technical solutions and incorporating research on unequal social structures. Because AI systems are embedded in social systems, technical solutions to bias need to be contextualized in their interaction with those larger systems. This series explores problems and solutions in making AI more just. The first talk is by Ben Green on Oct. 5, and future speakers include Alex Hanna. Talks will be archived on the Center’s YouTube Channel.
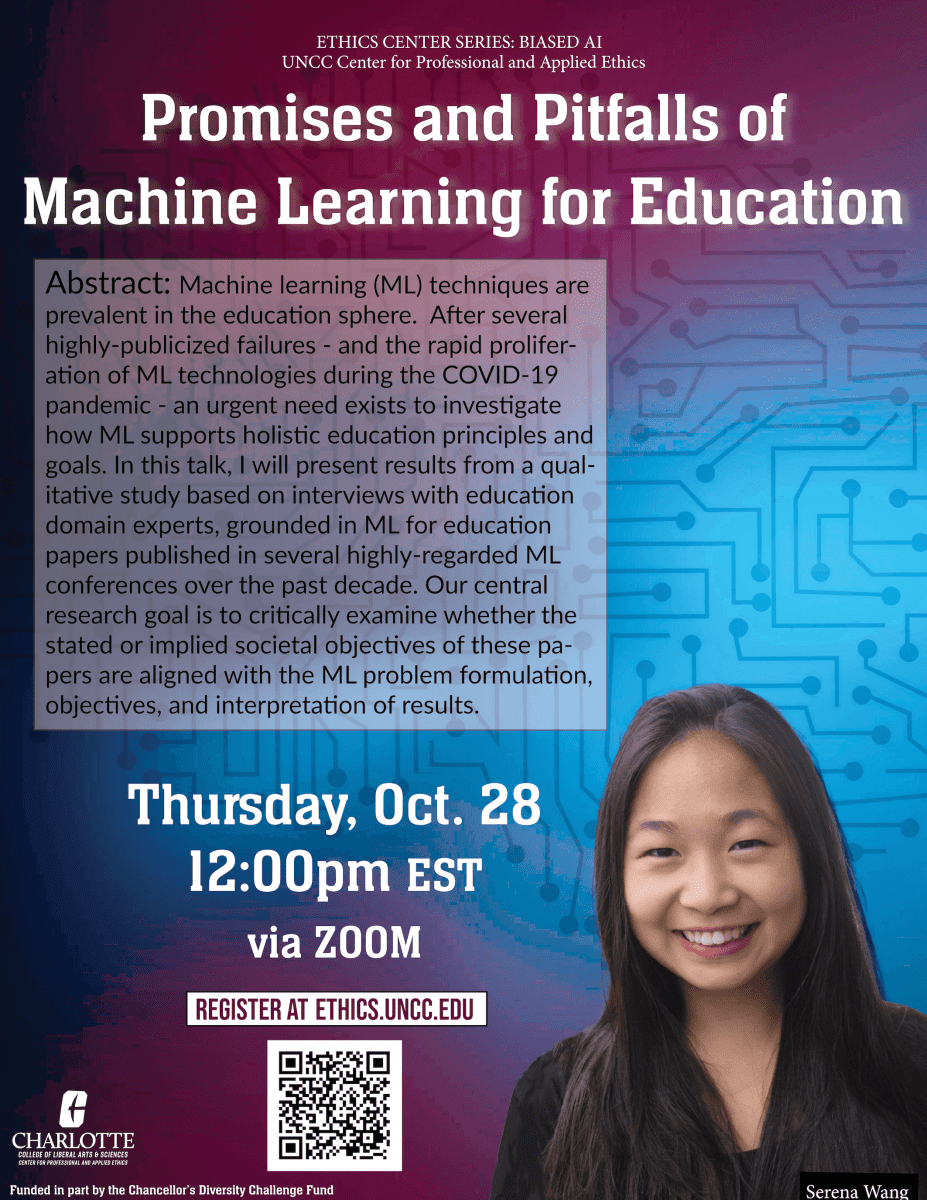